In this current post I have tried to compile most questions, discussions and queries I come across while consulting Data Science road maps with leaders and managers. I am hoping this compilation will add value to the other leaders and managers too who may have at some point wondered about them but didn’t get the opportunity to have those discussions. Many of you may have a better response or more exposure to some of the questions, I have tried to compile it based on the best knowledge I have and how I go about explaining them.
What I write about
- AI
- machine learning
- ml
- AI & Data
- management
- nlp
- AI adoption
- AI trust
- Data Science
- LLM
- bert
- cognitive analytics
- data analysis
- product management
- strategy
- text analytics
- transformer
- AI Development
- AI Optimization
- AI success
- API Tuning
- AWS
- Amazon
- Azure
- Azure Cognitive Services
- Azure Document Intelligence
- Batch Processing
- Cloud Solutions
- Conversational AI
- Data Processing
- Fair AI
- Flask
- Gemini
- John Nash
- John von Neumann
- OCR
- POS analysis
- Preprocessing
- Safe AI
- Sentiment Score
- Speed Optimization
- analytics
- bias
- brain
- business
- competition
- copilt
- core competency
- covid
- databricks
- ecommerce
- economics
- economy
- financial
- game theory
- gpt
- human
- hyper optimization
- investment
- leadership
- mlflow
- netflix
- predictive modelling
- product owner
- recommendation engine
- roi
- squad
- strategy. consultation
- streaming
- tableau
- vizualization
Monday, 19 June 2023
AI, ML, Data Science - frequent consultation advises to leadership
Please reach out, if you think you have a question or point that appears a lot during consultation and is worth discussed upon.
1. Should we build this product or capability in-house or get it from a vendor?
A vendor product will always be a generalized one to cut across as many businesses as possible as they thrive on repeat-ability. While when you build in-house you can make it more customized to a smaller set of use cases or scenarios and may be create a better differentiation.
So please ask yourself –
· When partnering with a vendor what role do I play? What stops the vendor from partnering with the business directly in future? What is my value addition, is there any risk I may become insignificant.
· What kind of team I have? If you have a great engineering team may be you want to do more stuffs in-house and keep a bigger piece of pie for yourself.
· What is my core capability? Is the capability needed in line with our core skill or is it something we want to learn, then maybe we should do it in house, or it is something we just want to get done, then may be the best way is to get a vendor involved.
2. We have created certain Analytics use cases. But we see several other teams also creating similar use cases.
Differentiation of analytics product or use cases are driven by each and combination of below –
a) Deep domain knowledge
b) Combination of data from different systems brought together on a dynamic big data system
c) Deep or mature algorithm applied
If your use cases are easy to replicate it’s most probably on a shallow data, with very general domain knowledge applied with basic Data Science techniques.
3. Are we using the kind of AI that is used for technologies like Self Driving car?
Yes and No. Internally all these technologies uses combinations of neural net and reinforcement learning. We also for different use cases have used variation of same and similar technologies. But technologies like self-driving car works on image or vision data, which we generally don’t do. Our use cases are mostly based on numerical, text and language processing data.
4. Vendor says their product is being used by Amazon. So should we go ahead and buy it?
May be it is being used by Amazon or any similarly big companies, but ask the vendor if their product is being used for a mission critical process or for some PoC or to store or process data like click-stream data which is not business critical. This makes all the difference, if the logos vendors show you are using the vendors technology for business critical projects or some non-critical processes.
5. We are showing the use case to the business but it’s not making much of an impact.
Story telling needs to be improved. Every analytics use case must be associated with a story that should end with the business making or saving money. If the story cannot relate how the engineering will improve the customer’s financial bottom-line, the customer business does not care about it, irrespective of how good the engineering is.
6. Now we have a data scientist in our team. Can we now expect more insights from our data?
Data Scientists alone cannot ensure project success. Data Engineers, Big Data and Cloud Infra Engineers are equally important part of the technical team. Without the infrastructure in place and data being stored in the infra in proper format, Data Scientists cannot do his or her magic.
7. We are finding very difficult to hire data scientists and big data developers.
Though there is no dearth of CVs, finding genuinely talented people with actual knowledge and production implementation knowledge is difficult. And among the few, most are already paid well and on good projects. So whenever a decision is taken to hire senior data science talents, a 6 month time frame should be kept in hand.
8. What is the difference between ML and AI?
Though you will find several answers to this in the internet one good way I have found to explain it to a business person, without the jargons is as below. By definition ML comes within the broader scope of AI. But to understand better and remember, Ai is something that is built to replicate human behavior. A program is called a successful AI when it can pass a Turing Test. A system is said to pass a Turing test when we cannot differentiate the intelligence coming from a machine and a human. ML is a system which you create to find pattern in a big data set that is too big for a human brain to comprehend. On a lighter note – if you ask a machine 1231*1156 and it answers it in a fraction of a second it is ML and if it pauses, makes some comment and answers after 5 mins, like a human, it is AI.
9. Why aren’t we using a big data Hadoop architecture but using RDBMS like MSSQL. Oracle.
RDBMS products like MSSQL, Oracle are still viable analytics products and are not replaceable by Big Data tools for many scenarios. Deciding on a data store or a data processing engine involves a lot of factors like ACID-BASE properties, type and size of data, current implementation, skill set of the technical team etc. So doing an analytics project does not make Hadoop or NoSQL product default.
10. Here is some data, give me some insight.
This is the first line of any failed initiative. A project which is not clear about the business problem it wants to solve is sure to fail. Starting on an analytics project without a clear goal in mind and for the sake of just adding a data science project to the portfolio and no road-map how this will eventually contribute to company goal, is a waste of resource and will only end in failure.
Sunday, 18 June 2023
Competition in the context of the new world order
Definition of competition has changed. And Elon Musk realized this long back. If you analyze any competitive business now you will somehow find one or few of the big tech 4 – (Facebook, Amazon, Microsoft, Google) involved in some way or the other. And what Elon knew was, his strongest competition will come from Google and Ubers of the world with their self-driving technology, rather than from GM or Ford.
And we know big tech 4 – (Facebook, Amazon, Microsoft, Google) strengths.
· Deep funds available to do experimental innovation. Significantly less pressure to go profitable.
· Army of engineers and scientists.
· Vast computer infra. So vast that a company can rent their unused infra and it can become one of world’s biggest business (read AWS)
So the big question is how do their competitors stay relevant and significant. They should be using what they have gathered over years of being in business i.e. tricks of trade or domain business knowledge. It can be years of building cars, manufacturing things or making software products.
So the strategy can be –
· Avoid direct competition with the tech giants. If the product is too generic in nature they will build it faster and better with their deep resources.
· Integrate data science closely with business products. Analytics should be out of the box and intuitive. If required collaborate with the tech giants’ offerings but never give away domain expertise.
· Enable data science teams with domain knowledge. Celebrate people who are domain experts and make them part of the data science team.
Sunday, 4 June 2023
Building the next Netflix
Recently I helped out a friend who is starting a video streaming gig to layout the roadmap on how he should go about developing the video content recommendation engine. Since I got good feedback, I thought of sharing the same with you. And you can apply the same principles to any recommendation, irrespective of the product.
So let’s assume you are also trying to build a video streaming company which will compete with the likes of Netflixes of the world. We have to come up with a complete road map of how the recommendation engine will be built from day 1 till you are a billion dollar company.
Here are our competitors –

Since we are a new business and we are in the business of recommending movies, we want to start off quickly.
The problems are –
o All our users are new. That is, we don’t have any history of individual users.
o And since our company is also new, we haven’t also still learned what kind of movies people of different age, gender etc. prefer to watch, so that we can recommend.
So as we collect more data let’s start with level 1 recommendation strategy.
· Level 1: Popularity. Recommending products is just based off of the popularity of each product. The first most simple and basic method is to recommend the most popular movie irrespective of user characteristic. This can be deployed when you don’t have any history to work on, you don’t know anything about your user, or you are not much concerned about personalization, or you want to quickly start off with something while you develop the other approaches.
Pros:
1. Simple and easy to implement.
2. Can work on shallow data
Cons:
1. Lacks personalization
So what we will do for our Startup is we will collect the best rated movies from imdb’s public data and recommend the best rated movies to everyone.

We keep doing this until we collect enough data on what kind of movies different type of people are watching. Then we want to handle some level of personalization, so let’s move to level 2.
Now few days has passed for our Startup and due to good work done by our Marketing team, we have had good number of people who have signed up and watched some movies. With these data in place we get trends like –
Female, Age – 30-40 -> Likes -> {Romantic Comedies, Julia Roberts}
Male, Age – 30-40 -> Likes -> {Action, Arnold Schwarzenegger}
Male, Age – 35-45 -> Likes -> {Animated from Pixar Production}
And not just that. We can also find trends like
On a weekend, afternoon, comedies or romance genre are watched more, while thrillers and horrors are watched more during night times.
So next time when a user logs in we may recommend him movie based on his demography matching trends like above. But we are not removing Level 1 strategy completely. We now will implement both Level 1 and Level 2 strategy with some weightage to each.
· Level 2: Classification model. Where we're going to use features of both the products and the users to make our recommendations. So this classification model is going to take in features about the user, features about the past purchases of that user, features about the product that we're thinking about possibly recommending, as well as potentially lots of other features that we can talk about.
Pros:
1. It can be very personalized
2. Can capture context
3. Works well in very limited historical data
Cons:
1. Works poorly when features are insufficient or poor in quality.
Now at our startup we have reached a pretty advanced level of personalization and everyone is happy. But let’s look at certain scenario of –
Male, Age – 30-40 -> Likes -> Action, Arnold Schwarzenegger
Male, Age – 35-45 -> Likes -> Animated from Pixar Production
We can assume the Pixar movies are mostly for children watching from their parents account. So these kind of problems can be either solved by asking more questions from Users during sign up. But there are only a certain number of questions you can ask and rest you have to understand from individual patterns. In cases like these, we can implement Level 3. Here we analyze trends like people who watch movies from Pixar also tend to like movies from Disney.
So we may find trends like below which are very tightly coupled, which we can use for our recommendation.
People who like {Pixar}-> also likes ->{Disney, Animation, Ice Age Franchise}
People who like {Avengers} ->also like -> {Marvel Studio, Batman Franchise}
Again we will implement Level 1, Level 2 and Level 3 together with combined weightages to get the best possible solution.
· Level 3: Collaborative filtering. This brings us to the idea of co-occurrence of purchases. Product recommendation is built on information like if a person bought this item, then they're probably also interested in some other item because we've seen lots and lots of examples in the past of people buying those pairs of items together. Maybe not simultaneously, at the same time, but in the course of their purchase histories.
Pros:
1. Works well even when past data is unavailable for the individual user.
2. Handles lack of feature or poor quality feature well.
Cons:
1. Complex to build
Congratulations, you are now successfully running a unicorn video streaming company with a great recommendation engine.
Please reach out to me if you need any technical clarification.
Until next time.
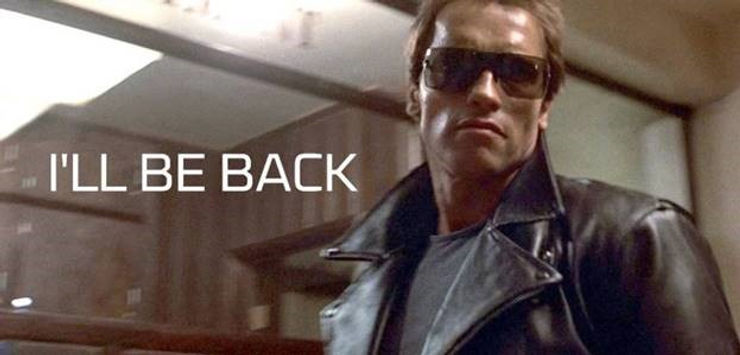
Subscribe to:
Posts (Atom)
- BIaaS plus Cloud Computing
- HADOOP – HOT INTERVIEW QUESTIONS - What is the responsibility of name node in HDFS?
- Artificial Intelligence - Man vs Machine
- BI on Cloud - Service overview and types of vendors
- Why Foreign Keys are now foreign to current DWBI applications
- Bit level SQL coding for database synchronization
- Future Trends in IT
- 10 THINGS TO KEEP IN MIND FOR AN ANALYTICS PROJECT
- What is Kerberos ?
- EMI – The dream killer – an Indian software engineer's perspective.